7 Most Common Mistakes in Data Analytics
Avoid these costly data analytics mistakes! Learn about the 7 most common pitfalls that can hinder your insights and how to avoid them.
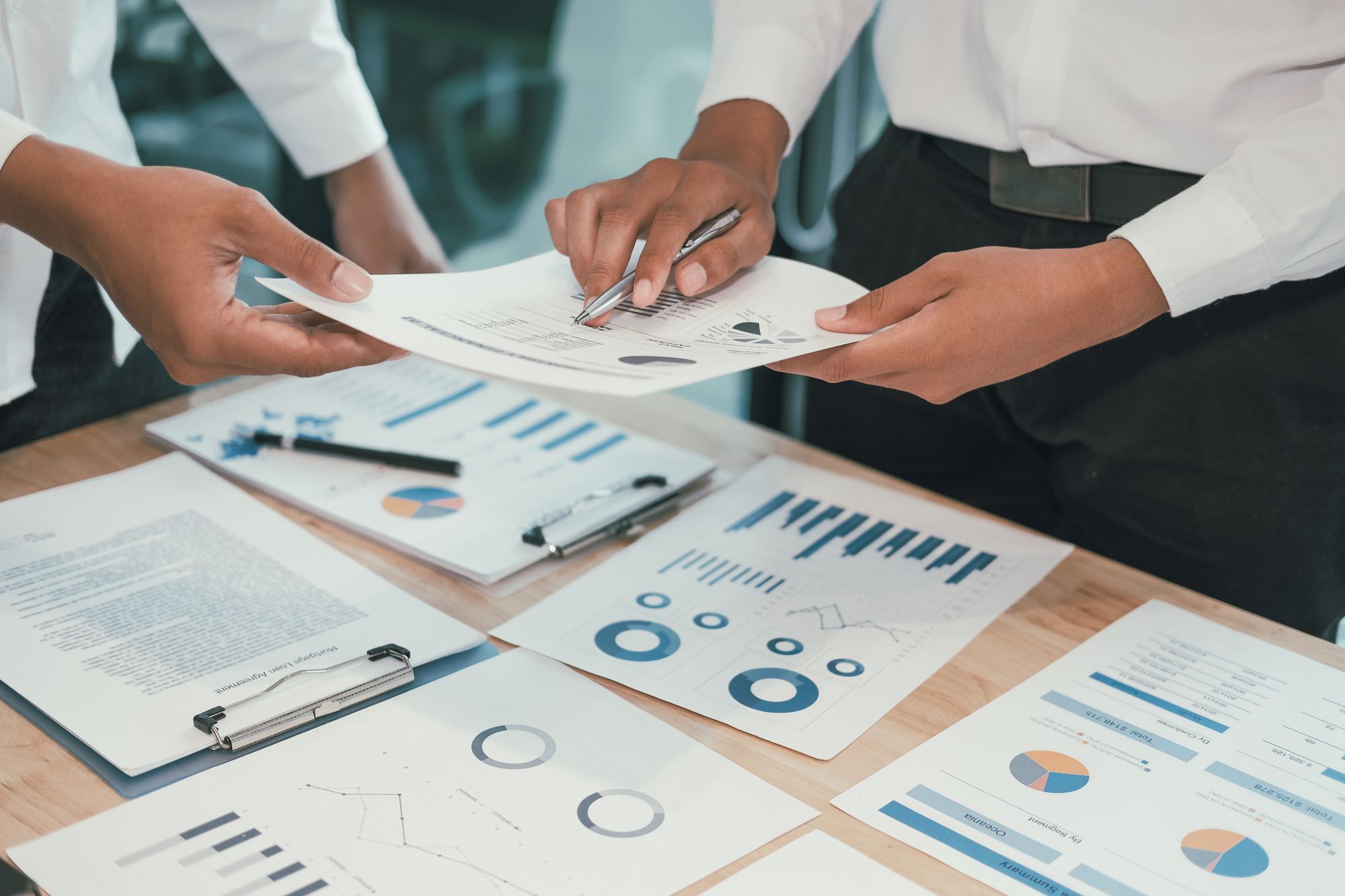
What is Data Analytics?
Data analytics is akin to a treasure hunt in the vast expanse of raw data, where the treasure is valuable insights that drive strategic business decisions. A subset of data science, it is a systematic approach to inspect, clean, transform, and model data with the objective of discovering useful information, drawing conclusions, and supporting decision-making.
Imagine standing before an uncharted jungle of unprocessed data points, with data analytics being your seasoned guide, leading you efficiently to the gems of insights hidden within. The nuanced landscape of data analytics comprises various advanced techniques, from basic data processing and statistical analysis to advanced machine learning, deep learning, and predictive modeling.
In this article, let’s take a closer look at data analytics to uncover the common pitfalls that can hinder a team and its stakeholders’ discovery of actionable insights, and find out a comprehensive solution for it.
Types of Data Analytics
Let's consider a thriving e-commerce business as a running example to understand the various types of data analytics:
- Descriptive Analytics: Going by the example of the e-commerce business, descriptive analytics might analyze sales data from the past year to identify the most popular products, peak shopping times, and trends in customer behavior. This forms the foundation of data-driven decisions by providing a clear view of past performance.
- Diagnostic Analytics: For instance, if the e-commerce business sees a sudden drop in sales, diagnostic analytics might uncover that a server outage during peak shopping hours was the culprit.
- Predictive Analytics: In our e-commerce example, predictive analytics could forecast future sales trends based on historical data, helping the business to optimize stock levels, adjust marketing campaigns, and anticipate peak sales periods.
- Prescriptive Analytics: Continuing with our e-commerce scenario, let's say predictive analytics foresees a tidal wave of demand for a new product based on market trends. Here's where prescriptive analytics leaps in, suggesting a strategic move like ramping up the inventory of this particular product, ensuring that when the wave hits, your business isn't just riding it out but surfing atop it, maximizing sales and customer satisfaction.
Each of these analytics types plays a crucial role in an organization's data strategy, enabling them to understand past performance, diagnose issues, predict future outcomes, and make data-driven decisions to drive success.
Why Data Analysis Matters
In our increasingly data-saturated world, data analysis has emerged as a vital pillar of any successful business, and here's why:
1. Informed Decision Making: Consider our e-commerce company example. Through the application of a data analytics platform, the company can identify top-performing products, pinpoint seasonal demand trends, and understand customer behaviors in terms of their social media preferences and more.
This wealth of knowledge guides strategic decision-making, from deciding which products to stock up on, to determining the optimal timing for promotional campaigns.
2. Efficiency: By examining data on various aspects of their operations, our e-commerce company can identify bottlenecks in their supply chain or inefficiencies in their website design that may be causing shopping cart abandonment.
Addressing these issues based on data analysis results in smoother operations, cost savings, and improved customer experience.
3. Enhanced Marketing Initiatives: By scrutinizing customer data, e-commerce data analytics can craft bespoke marketing strategies that harmonize with individual customer inclinations, thereby elevating customer involvement and boosting conversion rates.
For instance, if data analysis exposes that customers who frequently purchase electronic gadgets also exhibit a tendency to buy high-end headphones, the company can harness this insight to cross-promote these products.
This tactic not only bolsters customer satisfaction but also amplifies the efficacy of their marketing endeavors.
7 Common Mistakes in Data Analytics
As we navigate the vast ocean of big data analytics, it's crucial to avoid certain pitfalls that can compromise the effectiveness of our analysis.
1. No clear goal or objective
Embarking on a data analytics journey without a clear goal or objective is like a ship setting sail without a destination. Without a well-defined goal, data analytics efforts lack direction and fail to deliver actionable insights.
For instance, if the goal is to enhance customer retention, a more specific and measurable objective can significantly strengthen the analytics process. In our case, a well-defined goal would be to increase the number of customers who make repeat purchases within a specific timeframe, such as 4 months, by 10%.
2. Confirmation bias
Confirmation bias is a cognitive trap where one unintentionally seeks or interprets data in a way that confirms pre-existing beliefs or hypotheses, leading to skewed analysis.
For example, if our e-commerce firm believes that most of its customers prefer shopping on weekends, it might unconsciously lean towards data that supports this assumption while downplaying or disregarding contradictory information. Overcoming confirmation bias involves embracing objectivity, questioning assumptions, and being open to data that challenges our beliefs.
3. Incorrect benchmarks for comparison
Using incorrect benchmarks can lead to misguided business intelligence. If our e-commerce company, primarily dealing in electronics, compares its sales metrics with a fashion retailer, the analysis would be fundamentally flawed due to the difference in industry dynamics.
Benchmarking should ideally be carried out with competitors in the same industry (meaning that the e-commerce company should compare sales metrics to another e-commerce company with a similar business model).
4. Not enough context to present the results
Presenting results without adequate context can lead to misinterpretation of the data. Imagine our e-commerce firm witnessing a sudden spike in sales. Without context, this could be attributed to successful marketing campaigns.
However, DataGPT's dashboard, which provides unlimited drill-down of dimensions, might reveal that the spike was due to a price error leading to unusually high sales of a particular item. Contextualizing data helps in revealing the true story behind the numbers.
5. Unreliable data
Basing decisions on unreliable data is another common pitfall. If our e-commerce company uses data from a faulty tracking system, it may result in inaccurate customer behavior analysis.
Regular data audits, validation checks, and using reliable data sources are essential for maintaining data quality.
6. Poor visualization and communication of analysis
Even the best analysis can be rendered ineffective if not communicated properly.
If our e-commerce firm's data scientists discover an insightful trend but fail to present it in an easily digestible format, the decision-makers might miss out on taking timely action. DataGPT helps by providing easy-to-understand dashboards that clearly convey insights to decision-makers.
7. Misunderstanding metrics and KPIs
Misunderstanding or misaligning with the company's core KPIs can lead to wasted effort and resources.
For instance, if a marketing team in our e-commerce company focuses on driving website traffic while the company's goal is to increase conversion rates, the increase in website traffic might not contribute significantly to the company's success in achieving an increase in conversion rates.
Get Answers from Our Data in Seconds, Not Days, with DataGPT
Navigating the dynamic sphere of advanced analytics can be a challenging voyage, riddled with potential pitfalls. From setting clear objectives and mitigating confirmation bias to ensuring proper data visualization and understanding of key metrics, these practical insights can help us steer clear of common mistakes and capitalize on the transformative potential of data analytics.
However, even the most experienced data analysts need the right data analytics tools to fully harness the power of big data. DataGPT's intuitive analytics dashboard is designed to facilitate data-driven decision-making, offering a suite of features that translate into tangible benefits for businesses:
- Rapid, straightforward setup: DataGPT's Schema Builder shakes off the shackles of traditional BI and business analytics tools. Setup is completed in a single day, liberating your IT and engineering resources from the involved and lengthy procedures of typical data tools.
- Insights at lightning speed: Powered by our Lightning Cache Database model, DataGPT can query billions of rows of data in fractions of a second, automatically surface crucial insights, and unlock the power of instant data analytics.
- Clarity and objectivity in insights: DataGPT brings transparency to the forefront. There is no black box AI—instead DataGPT uses a completely transparent algorithm, so you can see the exact reason each segment was excluded or included.
- Flexible comparative analysis: The Real-Time DataGPT Analysis feature allows users to perform any comparative analysis by selecting any observation or comparison period and instantly seeing the results.
- In-depth Drill Downs: With Exhaustive Drill Downs, users can delve into any combination of dimensions with ease and accurately identify key action areas in mere seconds. This feature trims down the time to get answers and simplifies the user experience remarkably.
- Unified Dashboard: By consolidating all data and answers into a single, intuitive dashboard, DataGPT curbs 'dashboard paralysis' among business users, diminishing the time analysts spend on dashboard creation and data management, and enabling them to concentrate on higher-value tasks.
- Conversational AI Chatbot: DataGPT's chatbot experience acts as a personal data assistant. Empowering users to ask questions in everyday language, it elegantly translates complex analysis into easily comprehensible insights. Not only does it guide users towards the answers within their dashboard, but it is also leading the way in making sophisticated data insights accessible to business teams, setting a new industry standard.
In essence, DataGPT cuts down the time and complexity of daily analysis by 90%, reduces analyst backlog by 50%, and reinvents data culture with an impressive 85% business user adoption rate. It's a powerful BI&A solution that addresses the pain points of modern businesses, empowering users to make data-driven decisions with ease and efficiency.
Start your journey with DataGPT today.