AI Data Analysis: A Comprehensive Guide to Modern Analytical Techniques
Explore the transformative power of AI in data analysis and how conversational AI tools are reshaping business intelligence.
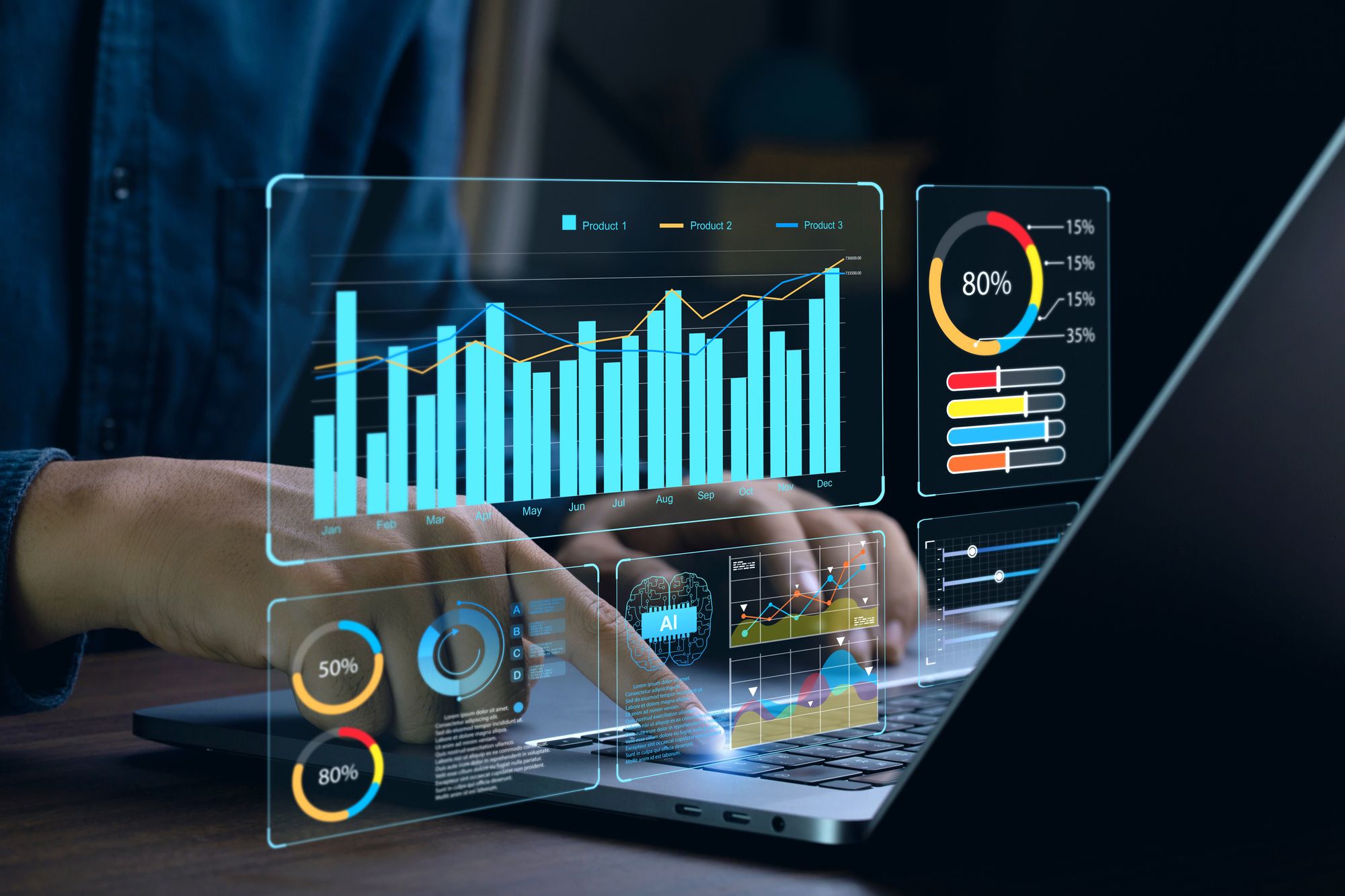
With innovative AI data analysis techniques, the field of business intelligence has witnessed many developments. OpenAI's ChatGPT, for instance, is a notable player in this arena.
Users can simply paste their data, often in CSV format, into ChatGPT and then ask specific questions about the dataset. They can also prompt ChatGPT to generate SQL queries, which can be executed on tools like Power BI.
However, every innovation has its boundaries. With tools like ChatGPT, users might face challenges like the restriction to analyze only small datasets and a potential inability to handle in-depth queries.
That's where DataGPT enters the picture. Instead of ChatGPT directly analyzing the data, our proprietary algorithms analyze it, and then DataGPT assists users in deriving insights through an engaging conversational interface.
This means you could effortlessly ask DataGPT something as broad as, "Why is revenue dropping?" and expect a coherent, insightful response.
DataGPT's proprietary caching technology supercharges query speed and cost on data warehouses, boosting efficiency. Furthermore, DataGPT is aware of the schema, product, and company specifics from the setup phase, ensuring tailored and accurate insights.
The bottom line?
Even if you lack technical prowess or SQL knowledge, you can pose any question in natural language, and DataGPT will delve into its advanced analysis algorithms to provide answers instantly.
In this article, let's look at how AI is revolutionizing the world of data analysis and how tools like DataGPT are democratizing access to valuable insights.
The State of AI in Business Intelligence
AI data analysis has emerged as an invaluable asset, integrating seamlessly into various BI workflows and bolstering their efficacy. Whether it's predictive analytics forecasting future trends, dynamic dashboards for data visualization, or using NLP for sentiment analysis, AI has elevated the capabilities of BI tools.
Several industry-leading data analytics software are leading this shift. For instance, Microsoft's Power BI harnesses the power of AI to transform raw data into actionable insights, offering intuitive visualization dashboards that allow businesses to recognize patterns and nuances at a glance.
Similarly, Tableau, another frontrunner in the BI space, uses AI to enhance its visualization capabilities, assisting businesses in drawing correlations and insights from vast datasets effortlessly.
Then there's MonkeyLearn, an AI platform that primarily uses NLP to process and analyze text data, perfect for tasks like sentiment analysis.
As more brands recognize the potential of these tools in identifying areas of optimization and brainstorming innovative solutions, their adoption becomes more mainstream. It's clear that the integration of artificial intelligence and business intelligence is not just a passing trend but a transformative force reshaping the business landscape.
Why AI Analytics Are Important
Industries like gaming generate a huge amount of user data from every interaction, transaction, and feedback, increasing the necessity for an efficient data analytics process.
This is where the advancements in AI data analysis come into play, revolutionizing the landscape for data analysts in various aspects of business development by:
- Speeding up data analysis processes: Tools like Power BI harness the power of generative AI, enabling quicker anomaly detection, which can be crucial in gaming analytics to flag unexpected player behavior or potential glitches.
Furthermore, AI aids in faster data cleaning, an otherwise time-consuming task. With tools like MonkeyLearn, analysts can swiftly sort through heaps of feedback, eliminating redundancies and inconsistencies. - Enhancing accuracy and efficiency: Data visualization tools like Tableau create intuitive dashboards, providing a bird's-eye view of vast datasets. Coupled with the prowess of GPT and other AI systems, these dashboards can be updated in real time, offering a pulse on evolving gaming trends.
The incorporation of machine learning algorithms ensures that as more data flows in, the system becomes better at reporting automation, minimizing human error, and maximizing data democratization. - Personalization and user-centric insights: Continuing with the same example, in the gaming industry, AI-driven tools can analyze player behavior to generate personalized insights.
This means that rather than a one-size-fits-all analysis, gaming companies can delve into individualized player experiences, making game enhancements that resonate with their audience.
In essence, AI's involvement in data analytics has not only streamlined processes but has also elevated the quality and depth of insights, proving invaluable for sectors that thrive on precision and personalization, such as gaming.
Challenges with Current AI Tools
Modern generative AI models like Code Interpreter showcase a promising direction in AI development but still face significant technical limitations when performing data analysis. While capable of answering direct queries, they struggle with abstract questions, such as, “Why is revenue dropping?” Furthermore, connecting to new data sources remains a technically expensive challenge, and these models are not designed to handle large datasets and data warehouse, making the integration process a cumbersome task that often requires the expertise of data engineers. These limitations constrain their applicability in complex data-driven environments
Similarly, the attempt to infuse Conversational AI capabilities into traditional BI solutions has exposed significant limitations in the quality of analysis that these solutions can provide. Though they may efficiently respond to direct queries, they are unable to address more intricate and abstract questions, such as understanding the underlying reasons for a drop in revenue. For traditional BI tools, the querying costs associated with these solutions can also be prohibitively high. Merely adding Conversational AI capabilities to existing BI tools does not necessarily translate into a more powerful or insightful analytical tool.
For both modern generative AI models and traditional BI tools, the lack of domain knowledge further limits their analysis capabilities, as they do not inherently understand the specific nuances and relationships within the dataset.
The disconnect between AI capabilities and real-world data requirements remains a barrier to achieving a seamless and sophisticated analytical experience.
Introducing DataGPT: The First Conversational AI Analyst
DataGPT is the world's first Conversational AI Analyst. This sophisticated software platform takes the concept of conversational AI to the next level by allowing users to interact with their data using plain English or any other human language.
Unlike other tools, DataGPT combines the power of Conversational AI and NLP to facilitate direct dialogue with your data. It's not just about using AI tools like ChatGPT on your data; it's a tailored system that understands data analytics in depth. Instead of struggling with complex Excel formulas or intricate databases, imagine having a detailed conversation with your data. You ask questions, no matter how complex, and DataGPT provides insights instantaneously, as though dialoguing with a highly skilled data analyst.
When you input a command or question, DataGPT employs a wide range of NLP algorithms and techniques to "understand" it, including entity recognition and tokenization. It then processes this input against your entire dataset to generate a comprehensible response.
Consider the advantages of this technology in the context of an e-commerce company:
- Speed: Conversational AI tools like DataGPT expedite processes. Rather than sifting through numerous graphs and tables, decision-makers can simply ask questions and receive answers within seconds, updating pricing or making critical inventory decisions swiftly.
- Accuracy: Human error is significantly reduced with specialized AI e-commerce data analytics tools like DataGPT. It can quickly provide an accurate overview, ensuring data-driven and precise decisions, compared to manual comparisons across different product categories.
- Economy: By cutting down the time-consuming tasks of data analysis, DataGPT not only accelerates decision-making but also reduces operational costs. It can present insights almost instantaneously, allowing for resource allocation to other crucial tasks.
In essence, DataGPT represents a paradigm shift in the landscape of e-commerce data analytics, offering a seamless blend of speed, accuracy, and economy. Unlike merely applying Conversational AI like ChatGPT to data, DataGPT is engineered as a comprehensive Conversational AI Analyst, enabling businesses to respond to market dynamics promptly and maintain a competitive edge. DataGPT is a fully autonomous chatbot with memory, capable of answering complex questions like "why did this happen?" and having any data-related conversation.
To truly grasp the transformative power of DataGPT in the realm of business intelligence, there's only one way — experience it for yourself. Book a demo today and dive into a world where data speaks your language.